Trust and transparency in science: An ongoing journey
Last month, I had the pleasure to give the opening keynote at the 18th edition of the International Digital Curation Conference (IDCC24) in Edinburgh. One of the many concerns of this age is the ability to trust information around us. Transparency is one way to promote trust, and in this sense the conference theme ‘Trust through Transparency’ struck me as both timely and relevant.
The context in which I have been thinking and talking about trust over the past years is of course related to my daily work at DANS, a national repository and centre of expertise.
Reusing data collected by others requires a lot of trust. We all know that there is an issue with trust in societies all over the world. Social media with its algorithms, fake news, and the rapid development of Artificial Intelligence (AI) play a big role in this diminishing trust. People simply do not know what or whom to trust anymore. Public trust in science is based on our hope and expectation that science will make our lives healthier, longer, more interesting, and therefore more pleasant.
What we also know is that public trust in science is an important parameter for assessing the impact of science. And there we touch upon a crucial point: trust in science is foundational for science to play its full role in our society. We need to do everything we can to make sure that scientific results find their way into society to help us deal with the immense societal challenges we are faced with today.
This means there is a big responsibility, also for the science sector itself, to do everything it can to make it as trustworthy as possible: this involves individuals as well as organisations. And it involves all stages of the scientific process: data gathering, processing and analysis, and the writing and publishing of papers.
However, just as any other part of society the science sector has its issues with trustworthiness. The massive data fraud case in 2011 by Diederik Stapel, a Dutch social psychologist who fabricated his data in at least 50 cases, is just one example. Cases like these are reported from all parts of the world and have probably always been around. With the arrival of AI, establishing the truth and creating trustworthiness becomes even more complex and challenging. If researchers share their data they can be inspected and re-used and Stapel would perhaps not have gotten away with his fraud for so long. Data sharing can be the basis for research verification and reproducibility, and it can also open up a path to broader collaboration.
Openness of data alone is not enough to create trust in the data, the focus on FAIR is an important step in the right direction. It says something about the care that was taken to make data available. This level of care given to a dataset, in turn partly defines its reusability. To be trusted by a re-user, the data not only need to be openly available, but also need to be trustworthy and in a way the perceived quality of the data is a proxy to their trustworthiness. Here the core elements of trust play a role: transparency, competence, integrity, consistency.
The FAIR principles and the many implementations of them, help us to improve the care that we give to a dataset. This defines what you could perhaps call the ‘technical’ quality of a dataset. But this is still not enough. I’ve noted before that a completely open dataset can be useless for others, if it is not FAIR enough and thus not reusable. A completely FAIR dataset does not give you guarantees about the quality of the research that led to these data. FAIR does not give you insight into the veracity of the data.
If we want to create trust in data, we need FAIR for the ‘technical’ data quality, to make sure that the data are findable, accessible, and interoperable. But for the R, the reusability, more is needed. FAIR needs to go hand-in-hand with scientific integrity, to assure the veracity of the data. The integrity of the research of which the data are the result, is an important dimension of its quality and thus of the trustworthiness of a dataset. Building trust will become more important with the growth of federated research infrastructures, the growing importance of multidisciplinary research to solve our problems, and last but certainly not least, the arrival of AI.
We are only in the middle of this journey towards trust in science and FAIR and open data. A lot of effort is currently going into the expansion of FAIR to other research outputs like software and semantic artefacts. We are developing metrics and tools to assess FAIRness, which comes with many challenges of its own. We are defining and operationalising what FAIR-enabling trustworthy research infrastructures and services could look like and how we could assess them. We are shaping Citizen Science and we are making our first steps towards new and more transparent and inclusive ways of assessing and rewarding researchers.
At DANS we try to contribute to a trustworthy data infrastructure with our Data Stations. Through our participation and coordination of European projects, like FAIR-IMPACT, and our active involvement in international organisations like CoreTrustSeal and the Research Data Alliance, we work with many partners around the globe towards Open Science, FAIR data and a trustworthy and transparent European data landscape.
Ingrid Dillo
Do you want to watch Ingrid Dillo’s keynote at the IDCC24? Click here to watch the video.
FAIR and Open data
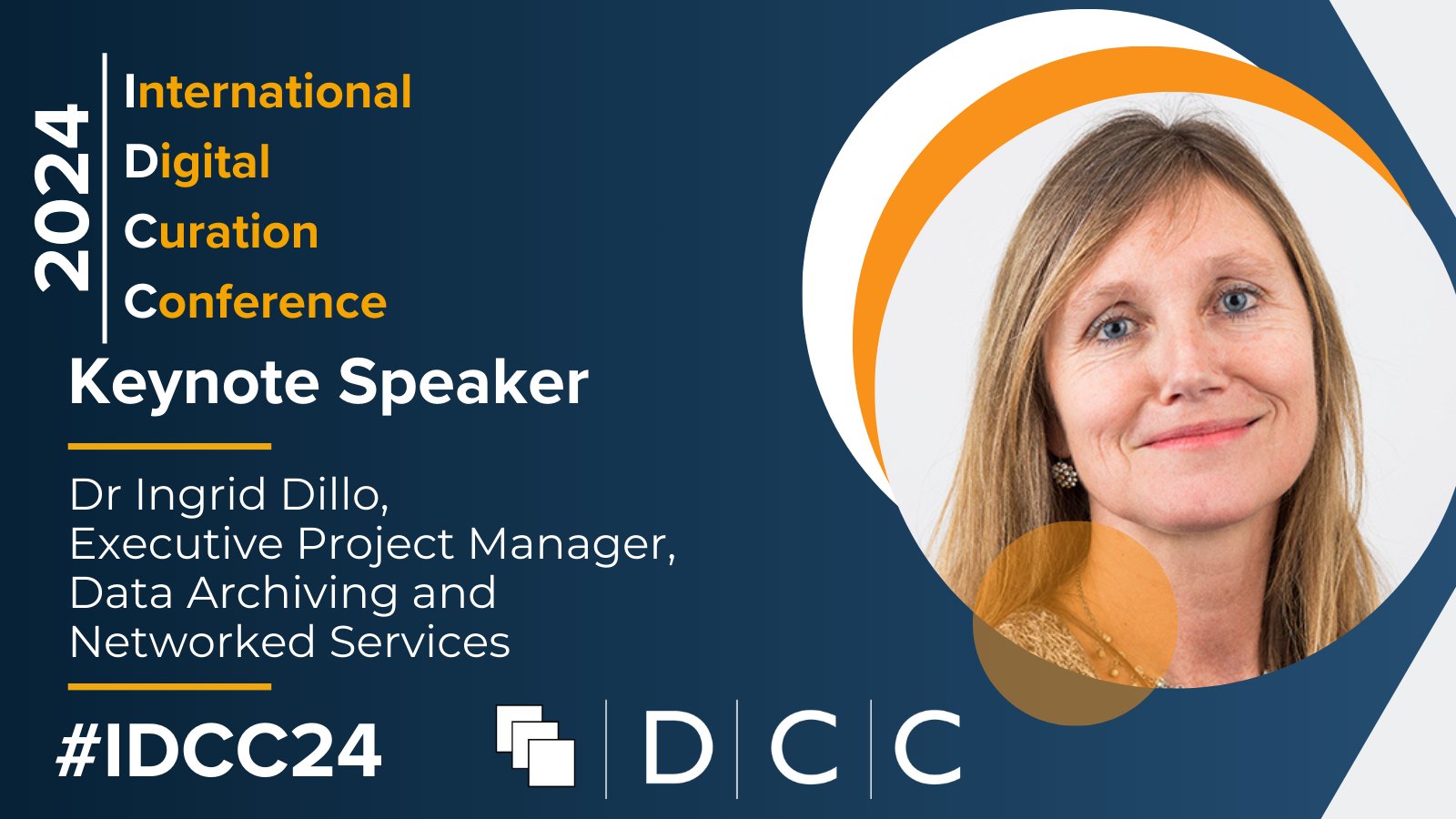